Continuous controls Monitoring
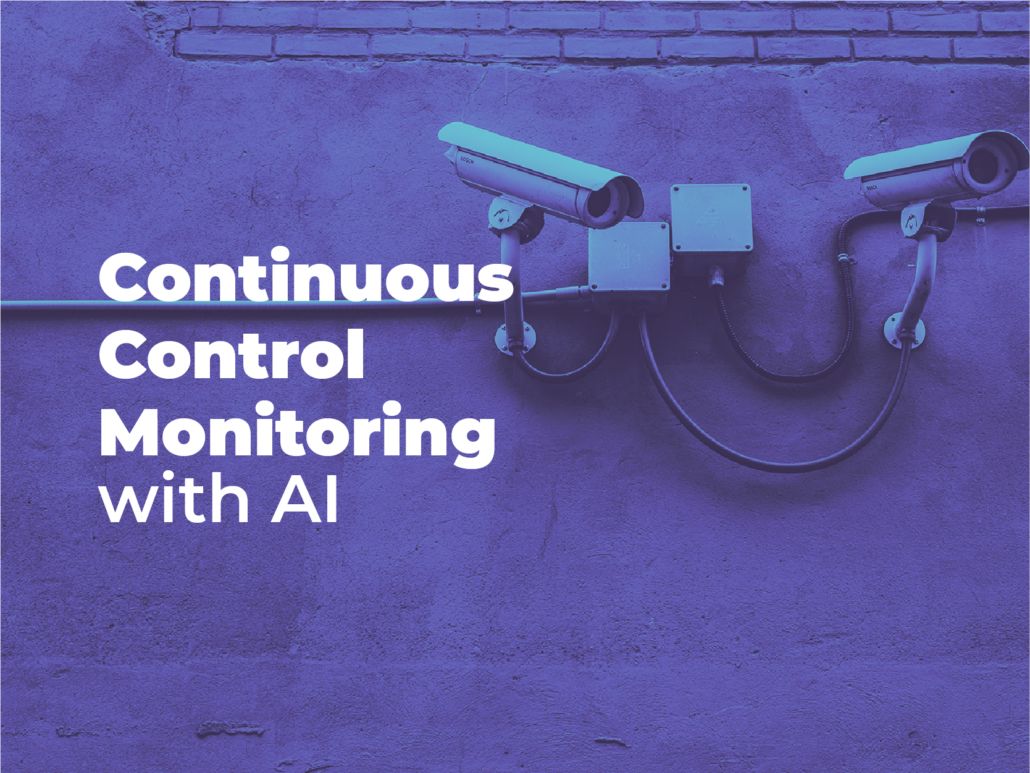
Continuous control monitoring (CCM) is a technology-based solution for constantly monitoring processes and leveraging sample-based testing methods for more cost-effective monitoring. CCM lowers audit costs by continuously monitoring transactional systems.
A simple Control Monitoring isn’t enough to keep things under control.
In a digital world, the control environments can not keep up with the difference in the ever-changing regulatory requirements and evolving risk dynamics. Reviewing thousands of processes, systems, and geographical locations, companies often find many overlapping and redundant controls and a significant manual effort to test and report the efficacy of the control environment. In addition, control rationalization and operationalization continuously keep the cost high.
Teams that keep an eye on risk must be aware of the rapid changes that might occur in an elegant setting on a minute-by-minute basis. Whatever the environment, whether it’s new product launches, financial information, or confidential customer information, it must be monitored and analyzed. Unfortunately, companies can’t keep up with this level of examination with only human resources. Unless a continual auditing process uses automation to achieve its goals, humans will make mistakes.
Therefore you see the old form of control monitoring is unacceptable, and there is a growing need for control definition to support new operational requirements and adapt to the hybrid work environment.
Control Monitoring with AI-based automation
Organizations need to plan for control transformation where internal control functions should include a common language of risk and control that offers clarity and centralizes tasks that can drive efficiency. With highly automated and real-time control environments, real-time identification of issues, rapid course correction, and optimal resource allocation for testing and monitoring controls allow management to focus on driving growth.
Process automation tools like RPA and unstructured data analytics with NLP and machine learning have a lot to offer. AI systems can support real-time monitoring of control and can automatically adapt to the exceptions and reduce human supervision. In addition, predictive insights can help continuous risk sensing.
Things to consider when selecting a control automation tool.
- How flexible it’s risk taxonomy and control framework?
- Does it support control libraries, the level of granularity for control definition, and how easy is it to create custom ones and integrate them with AI
- Can the AI adapt to ever-changing risk dynamics?
- What level of human supervision is required?
- What level of transparency does it provide, and does it supports AI explainability?
- Does it support risk-based control testing, and how flexible and the level of automation?
- Does it support an Open API & how well is it integrated with business applications?
- What is the level of flexibility it has for the operational model in a hybrid work environment?
- How well it supports governance and workflow around the 3-lines defense model?