Sentiment Analysis
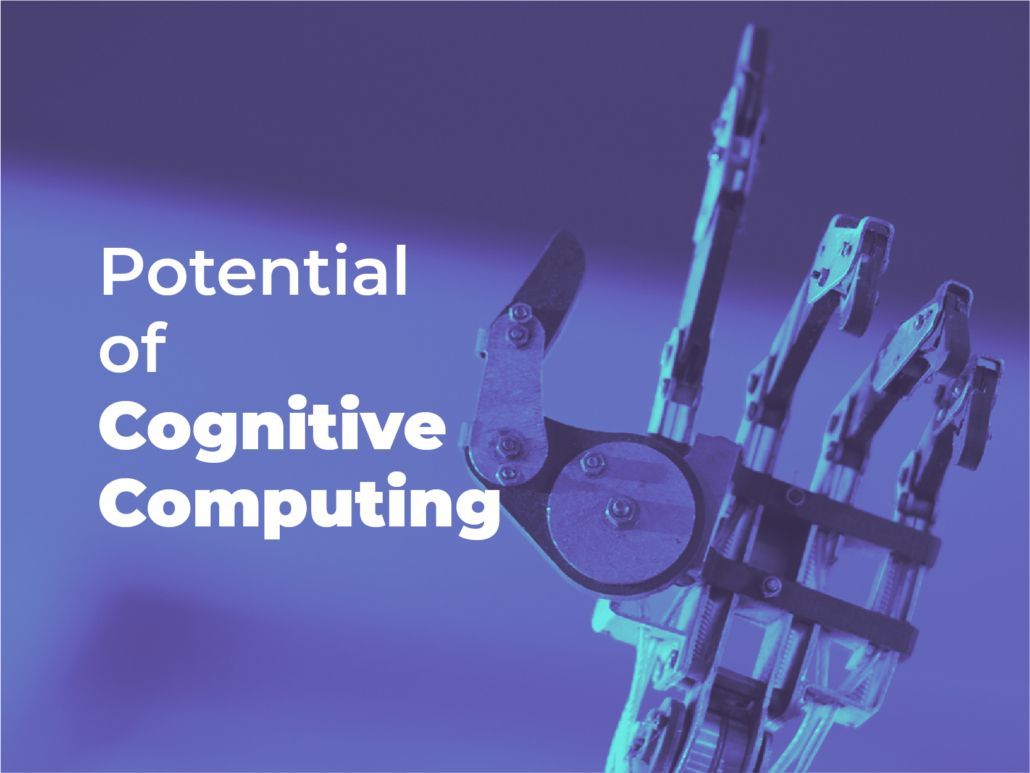
In the coming years, challenges posed by high-risk, low-frequency complaints could be addressed by cognitive computing. After all, firms in other industries have already begun using cognitive computing with artificial intelligence and analytical software to manage and glean insights from massive, complex data sets.
Cognitive technologies are capable of analyzing semantic features of text input, and therefore have the potential to decipher high-level concepts; interpret emotion and sentiment through specific target phrases, entities, and keywords; and recognize relationships and patterns — in essence, to understand natural language in context. When used with machine-learning functionality that automates analytical model building, these technologies also have the potential to learn iteratively and source insights without being programmed or instructed where to look. While these innovations are still under development, by combining natural-language-processing and machine-learning capabilities, engineers aim to design the next wave of cognitive technologies to compile and analyze data from a variety of unstructured sources, give advice and offer guidance, make hypotheses, and build cases upon evidence-based reasoning, among other capabilities.
For instance, a financial firm could teach a cognitive solution its definition of a complaint and then use it to identify high-risk language patterns and themes in complaint data across different mediums — including emails, call logs, and social media platforms — in near real-time. It could also use the technology to categorize complaints according to criteria such as issue type, level of possible severity, products and services mentioned in the complaint, employees, and locations mentioned in the complaint, possible financial impact to the firm, and even the likelihood of customer attrition.
Accurate, well-organized data would, of course, yield deeper insights from analytical activities. Most importantly, however, cognitive technologies could help financial firms eliminate current manual processes and support the consistent identification and categorization of complaints according to their level of risk, irrespective of their frequency.
Discovering the source of the issue
The complaint management process is a set of operations that used to handle complaints in organizations in order to resolve problems. A typical procedure for handling complaints are as follows

It is essential to have a sufficiently granular understanding of the complaint caseload by classifying it on severity type and type of complaint, which allows to conduct root cause analysis and to identify whether there are systemic issues that need to be addressed. From such regular reviews, it becomes possible to consider whether there are mitigations that can be put in place.
A root cause analysis using Cognitive technology can:
- identify the root cause, not just the symptom
- assist in creating a culture of identification and rectification before problems escalate
- helps the organization to focus on size, likelihood, and impact
Consider a scenario in which 1 million customers are inadvertently affected by an operational error that miscalculates the reward points for credit card usage over a period of several years. A fraction of those customers identify the error and complain directly to the bank, which receives just a few hundred complaints about the issue each month. One of those customers might call the bank and say to a representative, “I’m not receiving all my credit card points.” Another might call and say, “I should be getting cashback with every purchase, but I’m not.” Still, another might email the bank, insert “rewards” in the subject line, and write in the body, “I calculated my rewards balance for my credit card to be around 50,000 points, but I’m short.” And so on.
Through the application of cognitive technology, this firm could understand that the customers are complaining about the same issue, even though the language each customer uses is somewhat different. In addition, if the firm were to consider the issue “high-risk,” a cognitive solution could tag it as such and immediately escalate it for root-cause analysis and remediation. Given the relatively low number of complaints the firm received about the issue, its chances of finding the problem using traditional analytics would be limited.
Benefits
Potential benefits of using cognitive technologies for complaint management include:
Deeper Customer Insights
- Enhanced customer satisfaction, experience, and customer churn
- Improved insights into processes and product/service design
Early Identification of Complaints
- Early identification of complaint gives an opportunity for the organization to handle it efficiently
- Prevent the complaints ending with social media, regulator or ombudsman
Better Risk Management
- Improved data integrity by reducing the need for manual complaint categorization and data input
- Enhanced complaint insights and analytics
- Faster identification and remediation of issues
Direct Cost Reduction
- Increased efficiency across customer-facing platforms (call centers, chat functions, email intake, etc.)
- Increased efficiency across business lines and corporate complaint functions
- Reduced oversight and quality-assurance activities
- Quicker resolution
Every firm needs to ensure that it’s handling of customer complaints, and ombudsman cases is fit for purpose. There are significant reputational and customer satisfaction issues involved – not to mention significant costs.
Whether you are a consumer credit firm, a high street bank or an energy supplier, one fundamental principle is likely to be the same: you want to treat your customers fairly and achieve good outcomes. Automation leveraging AI can be used to reduce the number of complaints from occurring in the first place.
The technology exists that can interpret unstructured language, through voice or text, and identify not only what customers are wanting to achieve but also how they are feeling. This advanced technology opens new possibilities for triaging customers directly to people who are able to help. It also serves to prioritize channels of communication based on the individual customer’s needs, even identifying where vulnerability might become a concern.